Habitat maps are critical for effective management of the marine environment. However, map making can be time intensive, making it challenging to produce maps frequently enough to track environmental changes over time. Using artificial intelligence (AI) to automate part of the process can speed up map production. Our goal is to test multiple AI solutions for annotating underwater imagery, select the highest scoring approach, and integrate it with NCCOS’s existing map making process.
Why We Care
Part of NOAA’s mission is to provide reliable and timely scientific information about the location and condition of U.S. marine resources, including benthic habitats, which support sustainable fisheries and protect coastal communities. However, benthic habitat maps can require substantial manual effort to develop, making it challenging to produce them with the frequency needed to track environmental changes.
All of the steps in the map making process are highly technical: (1) acquiring remotely sensed imagery and underwater photographs, (2) annotating and extracting habitat information from underwater photographs, and (3) developing habitat maps and assessing their accuracy. NCCOS has improved the speed and repeatability of the first and last steps by implementing machine learning algorithms and leveraging the capabilities of uncrewed systems (UxS). However, step two is still largely a manual process, creating a time bottleneck and introducing unnecessary manual error and variability into the characterization process. This step is well suited for commercial off-the-shelf (COTS) AI solutions.
We will evaluate new COTS AI approaches for this step and integrate them as appropriate with existing NCCOS machine learning and UxS efforts to enhance NOAA’s ability to routinely develop accurate habitat maps in support of its marine stewardship mission.
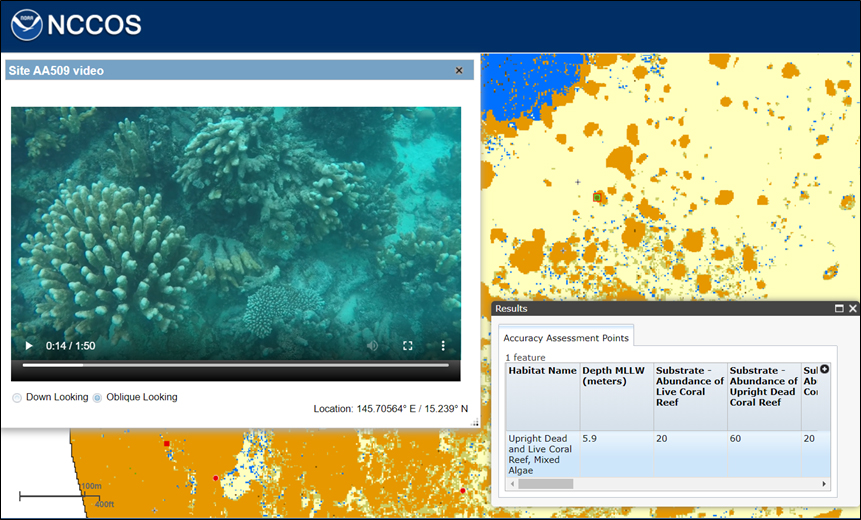
What We Are Doing
NCCOS will evaluate and compare suitable COTS AI options for automatically identifying and extracting habitat information from underwater imagery. The first step in this process will be to assess at least three COTS AI solutions that are both freely and commercially available. This preliminary analysis will likely include AI approaches developed by federal, academic, and private sector organizations, potentially including NOAA VIAME; University of California, San Diego (UCSD) CoralNet; Microsoft Azure AI; and Amazon Web Services Rekognition, as well as others.
These AI approaches will then be applied to existing, annotated underwater imagery to develop optimized AI habitat models. The cost and time required to develop optimized habitat models will be recorded for each AI approach. The accuracy of each optimized habitat model will be determined using a randomly chosen independent sample of annotations. Multiple performance metrics (e.g., overall accuracy, area under the curve, percent deviance explained, root mean square error) will be calculated to identify the most accurate and robust approach. These results will be compared to determine the optimal AI approach to extract habitat information from underwater imagery in support of the habitat characterization process.
Key findings from this research will be published in a final report. The report will include descriptions of: (1) the AI approaches tested, including their advantages and limitations, (2) the methods used to apply and optimize the AI habitat models, (3) the time, cost, and accuracy metrics for each AI approach, (4) the reason for selecting one, optimal AI approach, and (5) the process by which this optimal approach will be incorporated into NCCOS’s existing habitat classification process. After the report is published, NCCOS will begin integrating the highest ranked AI solution with its existing habitat characterization process.
The project was funded by NOAA’s High Performance Computing and Communications Program and will be conducted in partnership with NOAA’s Office of National Marine Sanctuaries. It will also be completed in coordination with NOAA’s Center for Artificial Intelligence and NOAA’s Fisheries Machine Learning and Computer Vision Community of Practice.
Benefits of Our Work
The highest scoring AI solution identified by the project team will be integrated with NCCOS’s habitat characterization process. The addition of AI will enable NCCOS to develop benthic habitat maps more frequently, improving NCCOS’s support of NOS and NMFS efforts to monitor and manage marine resources.